Buy vs build
Dec 2024
In the increasingly complex world of data management in the alternatives space, data extraction is arguably more important than ever. Rob Calder, enterprise sales executive at Canoe Intelligence, considers the benefits of third-party solutions over in-house
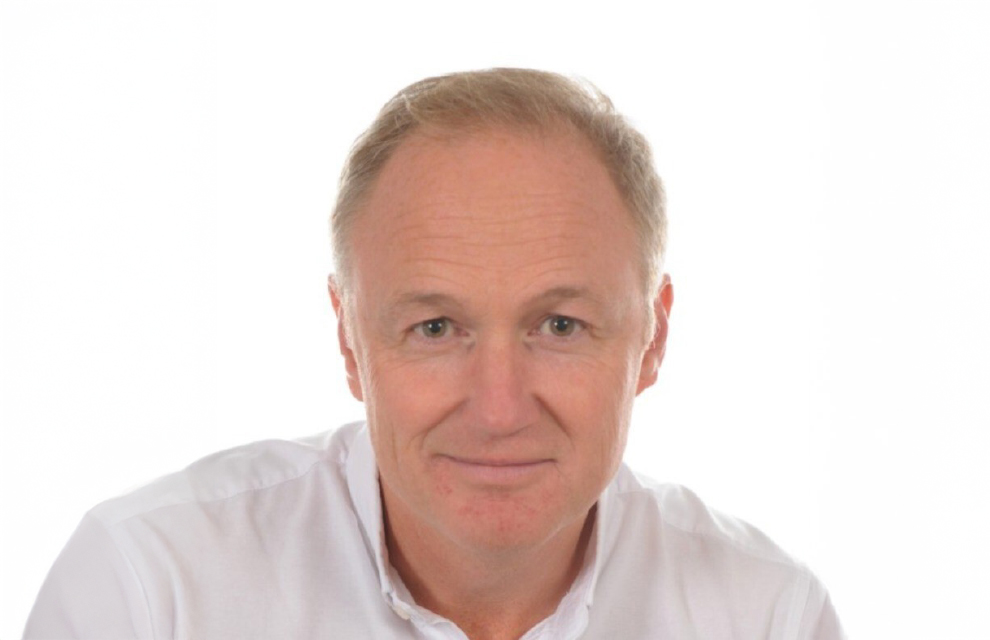
For any investor, precision and efficiency in data management are paramount. For alternative investors, the complexity of these assets and the lack of established standards make that precision and efficiency feel nearly impossible to attain, especially without the support of a technology solution.
Financial institutions navigating the intricate alts landscape often face the dilemma of whether to build an in-house solution or invest in an established technology like Canoe Intelligence. While the allure of total customisation may tempt some institutions to embark on the do-it-yourself (DIY) route, the hidden costs and risks associated with building an in-house solution are vast. While they may not offer the same level of tailoring, technologies designed for this purpose are already years ahead in terms of features and expertise — and will continue to be.
In the midst of the current artificial intelligence (AI) revolution, the promise of new generative AI tools is absolutely enticing. It is easy to believe a firm can leverage this tech to build its solution in-house, but not every challenge is suited for an AI solution. Other examples, like data extraction technology, are ideal use cases for AI but are also very difficult to build.
An ideal example
Using extraction as an example scenario, one can explore how and why an AI-based solution works to solve this challenge faced by alternative investors. It is no secret that alts funds are nuanced nor that different general partners (GPs) report differently — one data field can mean something entirely different between two different firms.
Some specifics that make this type of technology build complicated:
Mapping extraction rules: It is a complex, time-consuming undertaking to map out extraction rules for each new type of report. Given the lack of industry standards, it is highly likely that this extraction mapping continues to take place for each new alts fund a team invests in.
Relationship-dependent reporting processes: For each new GP the firm engages with, the team will potentially need to learn a new reporting structure and develop a new workflow to manage. With each new relationship, this need is likely to reappear, meaning the new fund onboarding process can never truly scale.
Closed-ended dataset: The true value of AI is found in the underlying model, and the model is ultimately dependent on its training data. If a firm’s extraction model is based solely on historical inputs from its own dataset, its potential will be limited by those inputs.
Where standardisation is lacking, industry expertise currently makes up the difference. However, without bringing AI into the equation, the expertise and efficiency that one person (or department) can manage has an upper limit. Thus, machine learning is paramount to scale data extraction from alternatives. A model can ingest the years of data history, built from thousands or millions of documents ingested, then synthesise and apply the learnings from that history to any new data points extracted. And, notably, those learnings can expand far beyond an individual or team’s own analytical capabilities — AI models excel at finding non-standard, edge cases that may have previously been inaccessible.
The big question — to build or to buy?
Even with a suitable use case for an AI application, and despite exponential advancements in AI capabilities, significant limitations remain that hinder the effectiveness and reliability of DIY solutions. As a starting point to build and train an AI model, a vast, accurate dataset is needed. Established providers will already have years, potentially even decades, of data to build a model upon.
Other limitations include the complexities of training an AI model to accurately extract data from each document format (and the options are diverse), the ongoing need for manual intervention to fine-tune algorithms (in perpetuity), and the inevitable challenge of maintaining compatibility with evolving regulatory requirements. As such, the apparent autonomy offered by in-house development may ultimately prove illusory, overshadowed by the hidden costs and risks inherent in building and maintaining proprietary extraction technology.
It is imperative for financial institutions to carefully consider the trade-offs between building and buying extraction technology, weighing the allure of autonomy and personalisation against the practical realities of scalability, reliability, and compliance.
The fallacy of building: Challenges and considerations
Managing alternative investments presents unique challenges, particularly in data extraction. While the final decision to buy versus build comes down to a number of factors unique to each business, it is important to call attention to the unavoidable cost across both scenarios — the cost of doing nothing. Manual extraction is tedious, time-consuming, and prone to human error — and the alts industry is quickly moving beyond manual.
For financial institutions considering building in-house solutions, several critical considerations will come into play:
Key person risk: Relying on a handful of individuals to develop and maintain proprietary extraction technology exposes a financial institution to key person risk. The departure of key personnel, whether due to turnover or unforeseen circumstances, could disrupt operations and compromise institutional knowledge. Such dependency on specific individuals can lead to significant setbacks and vulnerabilities in data management processes.
Resourcing: Establishing an in-house team for building and maintaining extraction technology requires substantial resources, both in terms of talent and infrastructure. From hiring specialised developers to investing in hardware and software, the upfront and ongoing costs can be considerable.
Moreover, ensuring the scalability and adaptability of in-house solutions demands continuous expenses for training, research, and development. Failure to scale efficiently means manual work is again required. As the demands for data extraction evolve, so do the resource requirements, stretching budgets and manpower thin.
Fragility of legacy tech: Integrating new extraction technology into existing legacy systems can be fraught with complications. In-house solutions may lack the interoperability necessary to seamlessly integrate with a financial institution's current infrastructure, opening up the potential for inefficiencies and technical debt. Moreover, the need to maintain compatibility with evolving technologies and regulatory requirements will add another layer of complexity, increasing the risk of operational disruptions and system failures.
Opportunity costs and maintenance burden: Building and maintaining solutions in-house diverts attention and resources away from core business objectives, potentially hindering an institution's competitiveness and growth. The focus on developing new features and the need for continuous maintenance may sideline critical initiatives and strategic endeavours, delaying time-to-market and impeding innovation.
Arguably, the opportunity costs associated with reallocating resources from revenue-generating activities to internal development projects, maintenance needs, or troubleshooting complexities can have long-term implications for financial performance and market positioning.
Innovation constraints: In-house development teams may lack the breadth of expertise available through third-party vendor teams who focus on these challenges daily. Context switching limits an institution's ability to innovate and adapt to emerging trends and technologies in the alternative investments landscape.
Moreover, the insular nature of development may lead to groupthink and stagnation, stifling creativity and hindering progress. By contrast, partnering with established vendors like Canoe provides access to diverse skills and perspectives, fostering innovation and driving continuous improvement.
Compliance risks: Compliance is paramount in the highly regulated world of finance. Developing in-house extraction technology may introduce compliance risks if the solution fails to meet regulatory requirements or industry standards.
Errors or inaccuracies in data extraction could lead to regulatory violations, fines, and reputational damage.
Ensuring compliance with ever-changing regulations adds another layer of complexity and potential liability for financial institutions, underscoring the importance of investing in proven, compliant technologies.
Unlocking the power of data with extraction technology
In the fast-paced world of alternative investments, precision and speed in data management can mean the difference between success and stagnation. Recognising the complexities inherent in this landscape, Canoe offers a solution purpose-built specifically for the nuances of alternative investments. With Canoe's extraction technology, financial institutions gain access to a comprehensive and reliable platform designed to streamline data extraction processes, providing unparalleled insights and efficiencies.
Superior Data Extraction: Canoe's extraction engine stands out for its unparalleled accuracy and efficiency. By leveraging cutting-edge approaches such as Natural Language Processing (NLP), text anchoring, spatial and coordinate recognition, meta-data analysis, machine learning pattern generation, and advanced table detection, Canoe ensures precise extraction of data from documents of any length or structure. Even challenging formats like floating tables are handled with ease, empowering clients to extract valuable insights from their investment documents effortlessly.
An ever-growing, shared intelligence: One of the key advantages of Canoe's extraction technology is its shared intelligence. As clients utilise the platform, Canoe continuously learns and improves based on collective usage. This shared knowledge allows clients to create bespoke data extraction patterns tailored to their specific needs in a matter of seconds.
With each interaction, Canoe's intelligence grows, enhancing its speed, accuracy, and processing rates. This ensures that clients always have access to the most advanced extraction capabilities, enabling them to stay ahead in an ever-evolving market.
Unparalleled access to data: Canoe's extraction engine empowers clients to unlock the full potential of their data. In addition to extracting required data elements for reconciliation, clients can delve into the rich historical documents that were previously inaccessible due to capacity constraints. Put simply, those edge case analyses are not so edge with Canoe as a partner.
This comprehensive dataset can be easily accessed and utilised for data science projects, deeper analytics, and informed decision-making.
By harnessing the power of Canoe's extraction technology, clients gain a competitive edge in the marketplace, driving innovation and growth.
Conclusion
In a landscape where data is king, your extraction technology should reign supreme. Consider your confidence level when it comes to the complexity of what an internal build entails, as well as how fast you would have to get there to beat the market.
No matter how many resources you have on hand, there will be a build time where you will continue in the inefficient experience.
Add in the open-ended expense of a build, likely to be larger than expected on a fixed basis and continuously growing with maintenance and development needs — and you have quite a sunk cost on your hands. Fortunately, there are no fixed costs with tech solutions as fees are typically based on usage.
With superior data extraction capabilities, ever-growing shared intelligence, and unparalleled access to data, Canoe empowers financial institutions to unlock new opportunities and drive success in the world of alternative investments.
To learn more about how Canoe can transform your data management processes, contact us today.
Financial institutions navigating the intricate alts landscape often face the dilemma of whether to build an in-house solution or invest in an established technology like Canoe Intelligence. While the allure of total customisation may tempt some institutions to embark on the do-it-yourself (DIY) route, the hidden costs and risks associated with building an in-house solution are vast. While they may not offer the same level of tailoring, technologies designed for this purpose are already years ahead in terms of features and expertise — and will continue to be.
In the midst of the current artificial intelligence (AI) revolution, the promise of new generative AI tools is absolutely enticing. It is easy to believe a firm can leverage this tech to build its solution in-house, but not every challenge is suited for an AI solution. Other examples, like data extraction technology, are ideal use cases for AI but are also very difficult to build.
An ideal example
Using extraction as an example scenario, one can explore how and why an AI-based solution works to solve this challenge faced by alternative investors. It is no secret that alts funds are nuanced nor that different general partners (GPs) report differently — one data field can mean something entirely different between two different firms.
Some specifics that make this type of technology build complicated:
Mapping extraction rules: It is a complex, time-consuming undertaking to map out extraction rules for each new type of report. Given the lack of industry standards, it is highly likely that this extraction mapping continues to take place for each new alts fund a team invests in.
Relationship-dependent reporting processes: For each new GP the firm engages with, the team will potentially need to learn a new reporting structure and develop a new workflow to manage. With each new relationship, this need is likely to reappear, meaning the new fund onboarding process can never truly scale.
Closed-ended dataset: The true value of AI is found in the underlying model, and the model is ultimately dependent on its training data. If a firm’s extraction model is based solely on historical inputs from its own dataset, its potential will be limited by those inputs.
Where standardisation is lacking, industry expertise currently makes up the difference. However, without bringing AI into the equation, the expertise and efficiency that one person (or department) can manage has an upper limit. Thus, machine learning is paramount to scale data extraction from alternatives. A model can ingest the years of data history, built from thousands or millions of documents ingested, then synthesise and apply the learnings from that history to any new data points extracted. And, notably, those learnings can expand far beyond an individual or team’s own analytical capabilities — AI models excel at finding non-standard, edge cases that may have previously been inaccessible.
The big question — to build or to buy?
Even with a suitable use case for an AI application, and despite exponential advancements in AI capabilities, significant limitations remain that hinder the effectiveness and reliability of DIY solutions. As a starting point to build and train an AI model, a vast, accurate dataset is needed. Established providers will already have years, potentially even decades, of data to build a model upon.
Other limitations include the complexities of training an AI model to accurately extract data from each document format (and the options are diverse), the ongoing need for manual intervention to fine-tune algorithms (in perpetuity), and the inevitable challenge of maintaining compatibility with evolving regulatory requirements. As such, the apparent autonomy offered by in-house development may ultimately prove illusory, overshadowed by the hidden costs and risks inherent in building and maintaining proprietary extraction technology.
It is imperative for financial institutions to carefully consider the trade-offs between building and buying extraction technology, weighing the allure of autonomy and personalisation against the practical realities of scalability, reliability, and compliance.
The fallacy of building: Challenges and considerations
Managing alternative investments presents unique challenges, particularly in data extraction. While the final decision to buy versus build comes down to a number of factors unique to each business, it is important to call attention to the unavoidable cost across both scenarios — the cost of doing nothing. Manual extraction is tedious, time-consuming, and prone to human error — and the alts industry is quickly moving beyond manual.
For financial institutions considering building in-house solutions, several critical considerations will come into play:
Key person risk: Relying on a handful of individuals to develop and maintain proprietary extraction technology exposes a financial institution to key person risk. The departure of key personnel, whether due to turnover or unforeseen circumstances, could disrupt operations and compromise institutional knowledge. Such dependency on specific individuals can lead to significant setbacks and vulnerabilities in data management processes.
Resourcing: Establishing an in-house team for building and maintaining extraction technology requires substantial resources, both in terms of talent and infrastructure. From hiring specialised developers to investing in hardware and software, the upfront and ongoing costs can be considerable.
Moreover, ensuring the scalability and adaptability of in-house solutions demands continuous expenses for training, research, and development. Failure to scale efficiently means manual work is again required. As the demands for data extraction evolve, so do the resource requirements, stretching budgets and manpower thin.
Fragility of legacy tech: Integrating new extraction technology into existing legacy systems can be fraught with complications. In-house solutions may lack the interoperability necessary to seamlessly integrate with a financial institution's current infrastructure, opening up the potential for inefficiencies and technical debt. Moreover, the need to maintain compatibility with evolving technologies and regulatory requirements will add another layer of complexity, increasing the risk of operational disruptions and system failures.
Opportunity costs and maintenance burden: Building and maintaining solutions in-house diverts attention and resources away from core business objectives, potentially hindering an institution's competitiveness and growth. The focus on developing new features and the need for continuous maintenance may sideline critical initiatives and strategic endeavours, delaying time-to-market and impeding innovation.
Arguably, the opportunity costs associated with reallocating resources from revenue-generating activities to internal development projects, maintenance needs, or troubleshooting complexities can have long-term implications for financial performance and market positioning.
Innovation constraints: In-house development teams may lack the breadth of expertise available through third-party vendor teams who focus on these challenges daily. Context switching limits an institution's ability to innovate and adapt to emerging trends and technologies in the alternative investments landscape.
Moreover, the insular nature of development may lead to groupthink and stagnation, stifling creativity and hindering progress. By contrast, partnering with established vendors like Canoe provides access to diverse skills and perspectives, fostering innovation and driving continuous improvement.
Compliance risks: Compliance is paramount in the highly regulated world of finance. Developing in-house extraction technology may introduce compliance risks if the solution fails to meet regulatory requirements or industry standards.
Errors or inaccuracies in data extraction could lead to regulatory violations, fines, and reputational damage.
Ensuring compliance with ever-changing regulations adds another layer of complexity and potential liability for financial institutions, underscoring the importance of investing in proven, compliant technologies.
Unlocking the power of data with extraction technology
In the fast-paced world of alternative investments, precision and speed in data management can mean the difference between success and stagnation. Recognising the complexities inherent in this landscape, Canoe offers a solution purpose-built specifically for the nuances of alternative investments. With Canoe's extraction technology, financial institutions gain access to a comprehensive and reliable platform designed to streamline data extraction processes, providing unparalleled insights and efficiencies.
Superior Data Extraction: Canoe's extraction engine stands out for its unparalleled accuracy and efficiency. By leveraging cutting-edge approaches such as Natural Language Processing (NLP), text anchoring, spatial and coordinate recognition, meta-data analysis, machine learning pattern generation, and advanced table detection, Canoe ensures precise extraction of data from documents of any length or structure. Even challenging formats like floating tables are handled with ease, empowering clients to extract valuable insights from their investment documents effortlessly.
An ever-growing, shared intelligence: One of the key advantages of Canoe's extraction technology is its shared intelligence. As clients utilise the platform, Canoe continuously learns and improves based on collective usage. This shared knowledge allows clients to create bespoke data extraction patterns tailored to their specific needs in a matter of seconds.
With each interaction, Canoe's intelligence grows, enhancing its speed, accuracy, and processing rates. This ensures that clients always have access to the most advanced extraction capabilities, enabling them to stay ahead in an ever-evolving market.
Unparalleled access to data: Canoe's extraction engine empowers clients to unlock the full potential of their data. In addition to extracting required data elements for reconciliation, clients can delve into the rich historical documents that were previously inaccessible due to capacity constraints. Put simply, those edge case analyses are not so edge with Canoe as a partner.
This comprehensive dataset can be easily accessed and utilised for data science projects, deeper analytics, and informed decision-making.
By harnessing the power of Canoe's extraction technology, clients gain a competitive edge in the marketplace, driving innovation and growth.
Conclusion
In a landscape where data is king, your extraction technology should reign supreme. Consider your confidence level when it comes to the complexity of what an internal build entails, as well as how fast you would have to get there to beat the market.
No matter how many resources you have on hand, there will be a build time where you will continue in the inefficient experience.
Add in the open-ended expense of a build, likely to be larger than expected on a fixed basis and continuously growing with maintenance and development needs — and you have quite a sunk cost on your hands. Fortunately, there are no fixed costs with tech solutions as fees are typically based on usage.
With superior data extraction capabilities, ever-growing shared intelligence, and unparalleled access to data, Canoe empowers financial institutions to unlock new opportunities and drive success in the world of alternative investments.
To learn more about how Canoe can transform your data management processes, contact us today.
NO FEE, NO RISK
100% ON RETURNS If you invest in only one asset servicing news source this year, make sure it is your free subscription to Asset Servicing Times
100% ON RETURNS If you invest in only one asset servicing news source this year, make sure it is your free subscription to Asset Servicing Times
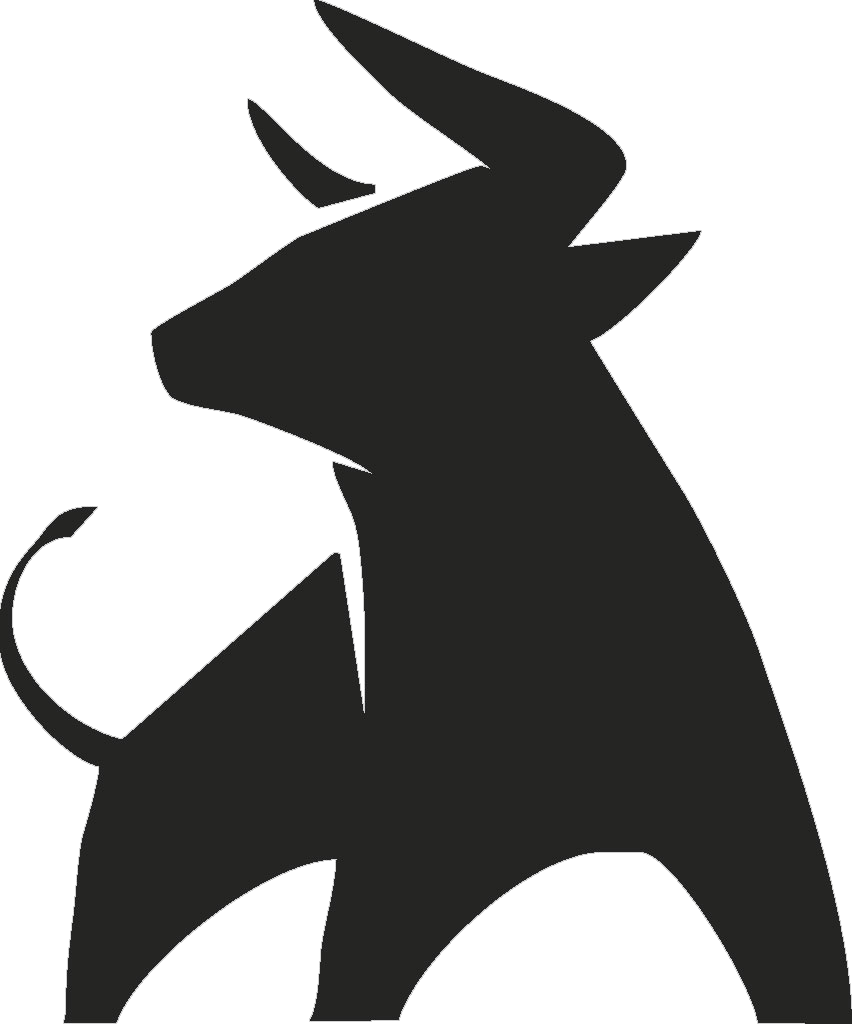